19 February 2024
PLEASE NOTE: The Viewpoints on our website are to be read and freely shared by all. If they are republished, the following text should be used: “This Viewpoint was originally published on the REVIVE website revive.gardp.org, an activity of the Global Antibiotic Research & Development Partnership (GARDP).”
The views and opinions expressed in this article are solely those of the original author(s) and do not necessarily represent those of GARDP, their donors and partners, or other collaborators and contributors. GARDP is not responsible for the content of external sites.
Antimicrobial resistance (AMR) is a severe reality that threatens human health. This resistance is a complex problem commonly associated with the overuse of antibiotics, which can lead to resistant bacteria selection and their further proliferation. In 2019, it was estimated that nearly 5 million deaths were associated with resistant bacteria,1 indicating that infections caused by resistant bacteria are one of the leading causes of death worldwide. Consequently, the development of novel approaches to treat resistant microorganisms is essential, especially for Gram-negative pathogens, as recently emphasized by the World Health Organization (WHO) and the European Centre for Disease Prevention and Control (ECDC).2
Bioinspired AMPs have been recognized as hopeful substitutes or additives to traditional therapies due to their multiple functions, including antimicrobial, antibiofilm, and immunomodulatory activities, in addition to reduced toxicity profiles.
Using bioinspired antimicrobial peptides (AMPs) in the fight against multidrug-resistant bacteria
One of the possibilities for controlling multidrug-resistant (MDR) bacteria is the use of bioinspired antimicrobial peptides (AMPs). Despite AMPs not being commonly related to low molecular mass drugs, which typically have an upper limit of around 900 Da, such peptide components are cationic and amphipathic molecules that can be isolated from various natural sources,3 designed in silico,4 or, in the case of bioinspired AMPs, generated through a combination of knowledge derived from nature enhanced by digital strategies.5 Bioinspired AMPs have been recognized as hopeful substitutes or additives to traditional therapies due to their multiple functions, including antimicrobial, antibiofilm, and immunomodulatory activities, in addition to reduced toxicity profiles.6 Despite natural AMPs being remarkable compounds found in six life kingdoms,6 several challenges need to be overcome to transform these compounds into pharmaceuticals. Unfortunately, some natural AMPs may have several limitations, including difficulties in purification processes, proteinase susceptibility, and potential for unspecific activities that reduce safety, high aggregation factors, and elevated production costs.7 Such challenges can make their clinical application problematic, including metabolic instability and toxicity.
The bioinspiration strategy assumes that nature knows better and that digital biology can transform it into something beneficial.
Using in silico methods to develop AMPs
In this view, the design of bioinspired AMPs focuses on utilizing in silico strategies to maintain the essence of natural AMPs9 and avoid the problems previously described that impede their use as commercial drugs.8,10 In summary, the bioinspiration strategy assumes that nature knows better and that digital biology can transform it into something beneficial. To design a novel bioinspired AMP, the structural profile, physicochemical properties, and biological activities of natural templates, including possible molecular targets, must be well explained. To develop a therapeutic candidate, a computational approach must redesign the natural AMPs, whilst retaining their fundamental properties and adding extra features such as bioavailability and human plasma stability. AMPs have an enormous amount of information stored in databanks, such as the 20-year-old Antimicrobial Peptide Database, which contains data on antibacterial activities, toxicity, and salt tolerance.11 This information is essential for bioinspired peptide design. Moreover, to be securely administered in the human body, bioinspired AMPs must exhibit high specificity.7 Importantly, all amino acid residues that are not essential for activity must be removed to reduce production costs.
The use of machine learning as an in silico method to develop AMPs
This is not an easy task, but several bioinformatics tools have been developed to assist in the construction of bioinspired AMPs. Machine learning procedures have been successfully used in predicting therapeutic peptides.12,13 For the generation of bioinspired AMPs, deep learning methods seem to aggregate value with other in silico tools. Deep learning methods can assemble and integrate a large quantity of information in a nonlinear manner, establishing more connections among data points and, consequently, assembling and integrating more knowledge.14 Some successes have been achieved with such a strategy. Certain AMPs have been generated using various natural language processing models combined to design and identify AMPs, including sequence generative adversarial networks, bidirectional encoder representations from transformers, and multilayer perceptron.15,16 In one case, six AMPs were selected by AlphaFold2 structure prediction associated with molecular dynamic simulations. Intriguingly, these AMPs showed low homology with known AMPs, arguably representing a novel class of bioinspired AMPs.16 Another report describes a machine-learning pipeline that mined an astonishing hundreds of billions of sequences in the entire virtual peptide library, consisting of six to nine amino acids, to identify potent AMPs.17 Three antimicrobial hexapeptides were identified by the pipeline with potent activities against a wide variety of clinically relevant MDR bacteria.16 These specific AMPs17 showed therapeutic efficacy in an animal model, negligible toxicity, and a low tendency to induce resistance. Finally, and not less importantly, machine learning techniques could also be used to avoid undesirable properties, such as hemolysis, as observed for bioinspired AMPs and anticancer peptides.18
The combination of multiple technologies will shed light on the discovery of novel antibiotics based on bioinspiration, potentially leading the fight against MDR bacteria into entirely new territory.
Perspectives and conclusion
In the short term, bioinspired AMPs still require endorsement and improvement. Nevertheless, digital biology and biotechnology have already greatly accelerated drug discovery and will likely continue to assist researchers in identifying promising candidates. Despite machine learning’s potential to accelerate and refine numerous aspects of AMP development, it demands high-quality datasets encompassing diverse peptide-positive characteristics. Machine deep learning has become increasingly valuable in AMP design, offering various benefits but addressing certain limitations. Amongst the benefits is the accelerated AMP discovery, including a high-throughput screening. Artificial Intelligence (AI) enables the rapid analysis of large datasets, facilitating the potential drug candidate’s selection. Moreover, target identification and validation could also be facilitated by AI. This technology helps to integrate and analyze diverse biological and chemical data sources, helping researchers identify and validate potential drug targets more efficiently. Machine learning algorithms can predict the biological activity and toxicity of potential AMP drug candidates.
Furthermore, AI-driven AMP drug discovery can improve resource allocation by prioritizing the most promising candidates. The number of unsuccessful trials was reduced, saving time and the cost of drug development. Otherwise, limitations are also observed for AI, e.g., data dependency. AI algorithms heavily rely on the quantity and quality of available data. Another problem consists of the black box model in the case of AMPs, especially due to the absence of AMP-specific targets. In that case, deep learning models, are often considered “black boxes” because their decision-making processes are not easily interpretable. Finally, and not less important, implementing AI in AMP discovery requires a significant initial investment in infrastructure and expertise. Nevertheless, the combination of multiple technologies will shed light on the discovery of novel antibiotics based on bioinspiration, potentially leading the fight against MDR bacteria into entirely new territory.
References
- Antimicrobial Resistance Collaborators. (2022) Global burden of bacterial antimicrobial resistance in 2019: a systematic analysis. Lancet. 399:629–655.
- Mancuso G, De Gaetano S, Midiri A, Zummo S, Biondo C (2023) The challenge of overcoming antibiotic resistance in carbapenem-resistant Gram-negative bacteria: “attack on titan”. Microorganisms. 27;11(8):1912.
- Zhu S, Weber DK, Separovic F, Sani MA (2021) Expression and purification of the native C-amidated antimicrobial peptide maculatin 1.1. J Pept Sci. 2021 Aug;27(8):e3330.
- Chen CH, Bepler T, Pepper K, Fu D, Lu TK (2022) Synthetic molecular evolution of antimicrobial peptides. Curr Opin Biotechnol. 75:102718.
- Porto WF, Irazazabal L, Alves ES, Ribeiro SM, Matos CO, et al. (2018) In silico optimization of a guava antimicrobial peptide enables combinatorial exploration for peptide design. Commun. 9, 1490.
- Silva O, de la Fuente-Nunez C, Haney E, Fensterseifer ICM, Ribeiro SM, et al. (2016) An anti-infective synthetic peptide with dual antimicrobial and immunomodulatory activities. Sci. Rep. 6, 35465.
- de Souza CM, da Silva ÁP, Júnior NGO, Martínez OF, Franco OL (2022) Peptides as a therapeutic strategy against Klebsiella pneumoniae. Trends Pharmacol Sci. 43(4):335-348.
- de Oliveira KBS, Leite ML, Cunha VA, Cunha NB, Franco OL (2023) Challenges and advances in antimicrobial peptide development. Drug Discov Today. 28(8):103629.
- Fernandes FC, Cardoso MH, Gil-Ley A, Luchi LV, da Silva MGL, et al. (2023) Geometric deep learning as a potential tool for antimicrobial peptide prediction. Front. Bioinform. 3:1216362.
- Wang Z, Yang N, Teng D, Hao Y, Li T, et al. (2022) Resistance response to arenicin derivatives in Escherichia coli. Microbiol. Biotechnol. 106, 211–226.
- Wang, G (2023) The antimicrobial peptide database is 20 years old: Recent developments and future directions. Protein Sci. 32(10):e4778
- Cherkasov A, Hilpert K, Jenssen H, Fjell CD, Waldbrook M, et al. (2009) Use of artificial intelligence in the design of small peptide antibiotics effective against a broad spectrum of highly antibiotic-resistant superbugs. ACS Chem Biol. 16;4(1):65-74.
- Liang W, Yu Q, Zheng Z, Liu J, Cai Q, et al. (2002) Design and synthesis of phenyl sulfide-based cationic amphiphiles as membrane-targeting antimicrobial agents against Gram-positive pathogens. J Med Chem 27; 65(20):14221-14236
- Söylemez ÜG, Yousef M, Kesmen Z, Büyükkiraz ME, Bakir-Gungor B (2022) Prediction of linear cationic antimicrobial peptides active against gram-negative and Gram-positive bacteria based on machine learning models. Appl. Sci. 12, 3631.
- Gupta R, Srivastava D, Sahu M, Tiwari S, Ambasta RK, at al (2021) Artificial intelligence to deep learning: Machine intelligence approach for drug discovery. Mol. Divers. 25, 1315–1360
- Cao Q, Ge C, Wang X, Harvey PJ, Zhang Z, et al. (2023) Designing antimicrobial peptides using deep learning and molecular dynamic simulations. Brief Bioinf. 19;24(2):bbad058.
- Huang J, Xu Y, Xue Y, Huang Y, Li X, et al (2023) Identification of potent antimicrobial peptides via a machine-learning pipeline that mines the entire space of peptide sequences. Nat Biomed Eng 7(6):797-810.
- Zakharova E, Orsi M, Capecchi A, Reymond JL (2022) Machine learning guided discovery of non-hemolytic membrane disruptive anticancer peptides. ChemMedChem 5;17(17):e202200291.
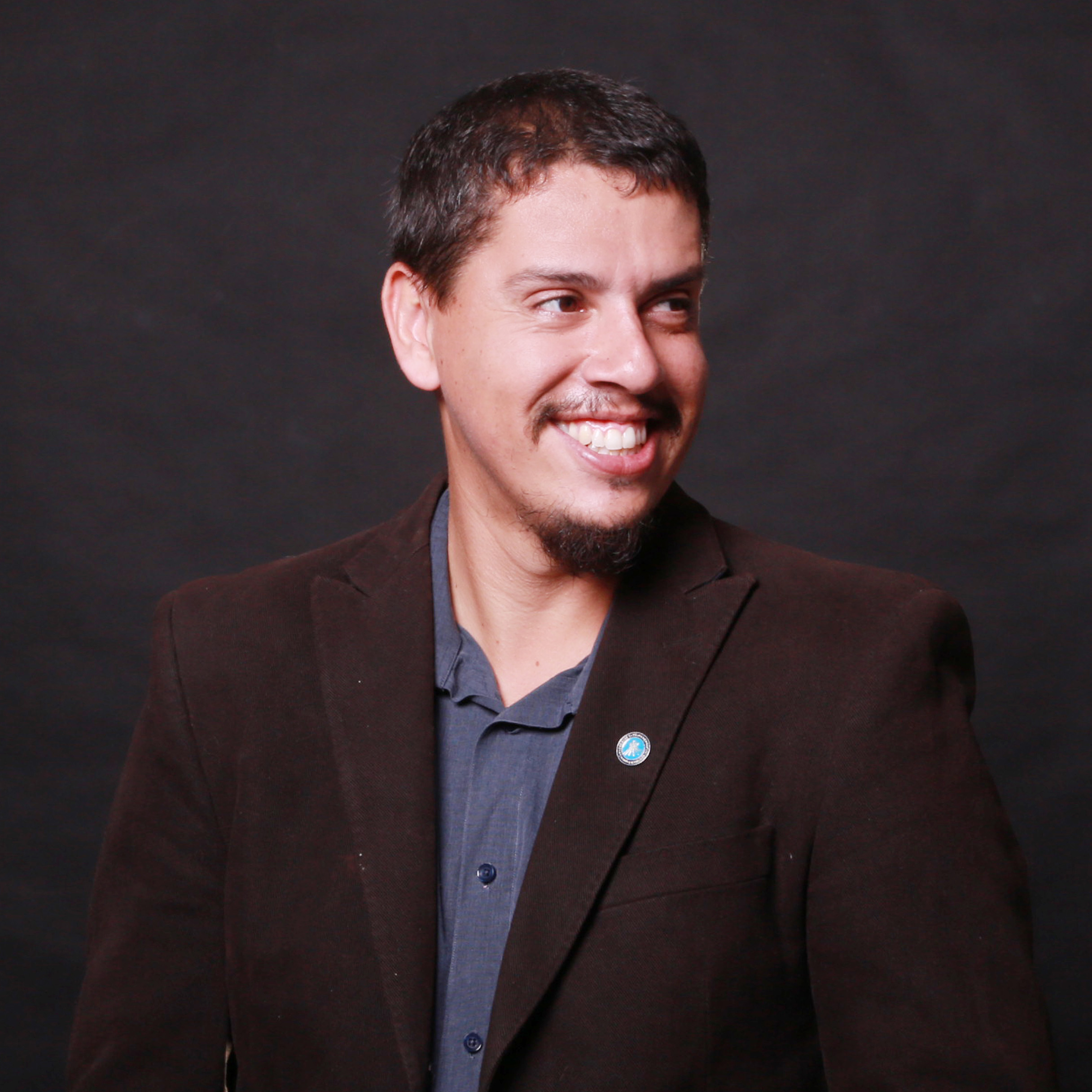
Octávio Luiz Franco is a researcher and a consultant to the Brazilian National Council for Scientific and Technological Development (CNPq). His work focuses on the development of bioproducts and bioprocesses, emphasising the control of pathogenic microorganisms. He obtained his first degree in Biological Sciences from the Federal University of Ceará and a PhD in Biological Sciences (Molecular Biology) from the University of Brasília.
Octávio worked on numerous missions in countless countries, including Australia, Israel, Portugal, and Cuba. He completed his first Sabbatical at the University of Wisconsin (USA) and the second at British Columbia University (Canada) in infectious disease control. He is a member of several societies, including the Brazilian Society of Biochemistry and Molecular Biology (SBBq) and the American Society of Microbiology (ASM).
The author declares that he does not have any relationships or affiliations that could be construed as a potential conflict of interest.