16 August 2023
PLEASE NOTE: The Viewpoints on our website are to be read and freely shared by all. If they are republished, the following text should be used: “This Viewpoint was originally published on the REVIVE website revive.gardp.org, an activity of the Global Antibiotic Research & Development Partnership (GARDP).”
According to the Antibiotic Resistance (AR) Threats Report released in 2019 from the United States’ Centers for Disease Control and Prevention (CDC), there are over 2.8 million antibiotic-resistant infections in the United States each year, leading to more than 35,000 deaths.1 In 2017, the World Health Organization (WHO) published its first priority pathogen list,2 which includes the drug-resistant ESKAPE pathogens: Enterococcus faecium, Staphylococcus aureus, Klebsiella pneumoniae, Acinetobacter baumannii, Pseudomonas aeruginosa, and Enterobacter species. These bacteria are the leading cause of hospital-acquired infections globally, calling for the need to discover new antibiotics.
Computer-aided methods have emerged as a promising approach to expedite drug design and discovery.3-6 For example, using computational algorithms, we have previously designed antibiotics effective in preclinical mouse models.6 Our group, along with collaborators, has also developed and validated a search algorithm to mine the human proteome for encrypted peptide antibiotics. This search was based on key physicochemical properties of antimicrobial peptides (AMPs), which are small molecules (8-50 amino acid residues in length) conserved throughout evolution and present in the innate immune systems of virtually all living organisms.7 More recently, we have expanded our efforts to mine the proteomes of archaic humans using machine learning coupled with experimental validation, introducing the concept of molecular de-extinction.8 Altogether, these recently developed computational approaches have dramatically accelerated our ability to discover new antibiotics, yielding numerous preclinical candidates.
Other recent advances in artificial intelligence (AI) and machine learning (ML) for antibiotic discovery
Since November 2022, the world has been taken by storm with the emergence of the AI chatbot ChatGPT, which is developed based on a deep-learning model called a large language model (LLM) that is trained on an enormous text database (i.e., the Internet) and can generate human-like sentences. Proteins are one of biology’s main biomolecules and are composed of an alphabet of 20 amino acids. Their structure and function are dictated by the ‘grammar’ embedded in the physicochemical properties of their amino acid sequences. Analogous to natural language models learning semantic and grammatical rules, LLM learns protein language to generate functional artificial sequences.9
Earlier this year, the ChatGPT-like language model ProGen was developed after being trained on 280 million protein sequences and controlled by property tags such as protein family, biological process, and cellular components that are largely available in public databases.9 This model was able to create two novel synthetic proteins related to lysozymes, which are small proteins found in blood, mucus, and hen eggs that kill bacteria by targeting their cell wall. The two proteins demonstrated comparable catalytic and bactericidal capabilities to hen egg white lysozyme (HEWL) but diverged by more than 30% from their natural sequences. This approach showed the possibility of employing a deep-learning-based language model to design de novo (from scratch) artificial proteins that are equally functional but distantly related in sequence space. This will likely speed up the search for new families of antimicrobial agents not necessarily found in nature.
Computational methods have already greatly accelerated antibiotic discovery and will likely continue to aid scientists in prioritizing those candidates that are most promising.
Furthermore, chemical space can also be considered a language, where the vocabularies are molecular fingerprints that suggest the structure-activity relationship (SAR) between the chemical structure and biological activity of a given molecule. For example, a fragment containing the hydrophilic R-OH group is a standard indicator of solubility.10 Newer architectures, such as message-passing deep neural networks, are able to learn their own task-specific representations using graph convolutions and achieve better molecular property prediction.11 Most recently, Liu et al.12 applied such approach based on their in vitro screening of ~7,500 molecules that inhibited the growth of A. baumannii and performed in silico predictions on the Drug Repurposing Hub13 for structurally new molecules with activity against this nosocomial pathogen.12 Through this method, they discovered abaucin, an antimicrobial compound with narrow-spectrum activity against A. baumannii.12
In addition to recent headlines made by ChatGPT and LLM, other computational tools have been developed based on neural network machine learning models to unveil the structure and mechanistic function of newly discovered natural therapeutics or de novo designed biologics.
For example, AlphaFold proved its importance in predicting protein structures.14 Prior to this advancement, painstaking crystallography,15 nuclear magnetic resonance (NMR)16 and cryo-electron microscopy (cryo-EM)17 experiments were necessary to determine protein structures. However, this constitutes only a minute fraction of the entirety of protein sequence space. AlphaFold incorporates novel neural network architectures and is trained on evolutionary sequence information in the form of multiple sequence alignment (MSA) and spatial information from 3D atom coordinates of homologous structures from Protein Data Bank (PDB). The first stage of the network called Evoformer enables a direct link between evolutionary information from MSA and spatial information from homologous structure templates. This is followed by a structure module that incorporates atomic details and a refinement process that fine-tunes the orientational correctness of the residues. AlphaFold shows its ability to predict protein structures to near experimental accuracy. Together with other recently developed structure prediction tools such as AlphaFold2, RoseTTAFold,18 and ColabFold,19 ML-based computational methods are posed to facilitate the understanding of protein structure and function. For example, Wong et al. combined AlphaFold2 with molecular docking simulations to predict drug-target interactions, which could potentially be used in antimicrobial discovery efforts.20
Unlike most antibiotics that currently reach the clinic, which are analogs of existing classes, some molecules developed by AI systems looked very different from compounds designed by medicinal chemists, opening new avenues for identifying entirely new antibacterial targets.
Challenges and outlook
Since AI and ML methods for drug design and discovery are relatively recent, no antibiotics generated by such methods have yet reached clinical trials. Nevertheless, encouraging results described in recent years have shown the power and potential of computers to design next-generation antimicrobials to combat the urgent problem of antibiotic resistance.
However, we still need to remain cautious about the content generated by machines, not only in the case of text output from ChatGPT regarding specialized knowledge such as medical queries, where the lack of accuracy is a concern,21 but also in the instance of generating potential drug candidates as discussed in this article. For example, not all artificial proteins created by ProGen were functional.9 Because most ML models are trained on public databases, the accuracy, robustness, and continuing expansion of the databases are critical for the future of AI for drug discovery. One strategy to improve the accuracy of generative AI in biomedicine is to focus on depositing more types of ‘metadata’ that can provide a more detailed and thorough account of the environmental (pH, temperature or pressure) and biochemical (chemical reactivity, thermodynamics or protein modification) context for a molecule.22 Another good research practice to implement in this emerging field is to generate robust in-house datasets containing validated data using experimentally standardized conditions.
In the short term, AI/ML-designed antibiotics still need further validation and improvement. However, computational methods have already greatly accelerated antibiotic discovery and will likely continue to aid scientists in prioritizing those candidates that are most promising. Also, unlike most antibiotics that currently reach the clinic, which are analogs of existing classes,23 some molecules developed by AI systems looked very different from compounds designed by medicinal chemists,24 opening new avenues for identifying entirely new antibacterial targets.
References
- Centers for Disease Control and Prevention (2019) Antimicrobial resistant threats in the United States, 2019.
- World Health Organization (2017) Prioritization of pathogens to guide discovery, research and development of new antibiotics for drug-resistant bacterial infections, including tuberculosis.
- Wong F, de la Fuente-Nunez C, Collins JJ (2023) Leveraging artificial intelligence in the fight against infectious diseases. Science. 381:164-170.
- Huang J, Xu Y, Xue Y, Huang Y, Li X, Chen X et al. (2023) Identification of potent antimicrobial peptides via a machine-learning pipeline that mines the entire space of peptide sequences. Nature Biomedical Engineering.
- Stokes JM, Yang K, Swanson K, Jin W, Cubillos-Ruiz A, Donghia NM et al. (2020) A Deep Learning Approach to Antibiotic Discovery. Cell. 180:688-702.e13.
- Porto WF, Irazazabal L, Alves ESF, Ribeiro SM, Matos CO, Pires AS et al. (2018) In silico optimization of a guava antimicrobial peptide enables combinatorial exploration for peptide design. Nature Communications. 9:1490.
- Torres MDT, Melo MCR, Flowers L, Crescenzi O, Notomista E, de la Fuente-Nunez C (2022) Mining for encrypted peptide antibiotics in the human proteome. Nature Biomedical Engineering. 6:67-75.
- Maasch JRMA, Torres MDT, Melo MCR, de la Fuente-Nunez C (2023) Molecular de-extinction of ancient antimicrobial peptides enabled by machine learning. Cell Host Microbe. 31: P1260-1274.E6.
- Madani A, Krause B, Greene ER, Subramanian S, Mohr BP, Holton JM et al. (2023) Large language models generate functional protein sequences across diverse families. Nature Biotechnology.
- Duvenaud DK, Maclaurin D, Iparraguirre J, Bombarell R, Hirzel T, Aspuru-Guzik A et al. (2015) Convolutional networks on graphs for learning molecular fingerprints. Advances in Neural Information Processing Systems. 28:2224-2232.
- Yang K, Swanson K, Jin W, Coley C, Eiden P, Gao H et al. (2019) Analyzing learned molecular representations for property prediction. Journal of Chemical Information and Modeling. 59:3370-3388.
- Liu G, Catacutan DB, Rathod K, Swanson K, Jin W, Mohammed JC et al. (2023) Deep learning-guided discovery of an antibiotic targeting Acinetobacter baumannii. Nature Chemical Biology.
- Corsello SM, Bittker JA, Liu Z, Gould J, McCarren P, Hirschman JE et al. (2017) The Drug Repurposing Hub: a next-generation drug library and information resource. Nature Medicine. 23:405-408.
- Jumper J, Evans R, Pritzel A, Green T, Figurnov M, Ronneberger O et al. (2021) Highly accurate protein structure prediction with AlphaFold. Nature. 569:583-589.
- Jaskolski M, Dauter Z, Wlodawer A (2014) A brief history of macromolecular crystallography. FEBS Journal. 281:3985-4009.
- Wüthrich K (2001) The way to NMR structures of proteins. Nature Structural Biology. 8:923-925.
- Bai X, McMullan G, Scheres SHW (2014) How cryo-EM is revolutionizing structural biology. Trends in Biochemical Sciences. 40:49-57.
- Baek M, Dimaio F, Anishchenko I, Dauparas J, Ovchinnikov S, Lee GR et al. (2021) Accurate prediction of protein structures and interactions using a three-track neural network. Science. 373:871-876.
- Mirdita M, Schütze K, Moriwaki Y, Heo L, Ovchinnikov S, Steinegger M (2022) ColabFold: making protein folding accessible to all. Nature Methods. 19:679-682.
- Wong F, Krishnan A, Zheng EJ, Stärk H, Manson AL, Earl AM et al. (2022) Benchmarking AlphaFold-enabled molecular docking predictions for antibiotic discovery. Molecular Systems Biology. 18:e11081.
- Zhavoronkov A (2023) Caution with AI-generated content in biomedicine. Nature Medicine. 29:532.
- Bender A, Cortés-Ciriano I (2021) Artificial intelligence in drug discovery: what is realistic, what are illusions? Part 1: Ways to make an impact, and why we are not there yet. Drug Discovery Today. 26:511-524.
- World Health Organization (2022) 2021 Antibacterial agents in clinical and preclinical development: an overview and analysis.
- Arnold C (2023) Inside the nascent industry of AI-designed drugs. Nature Medicine.
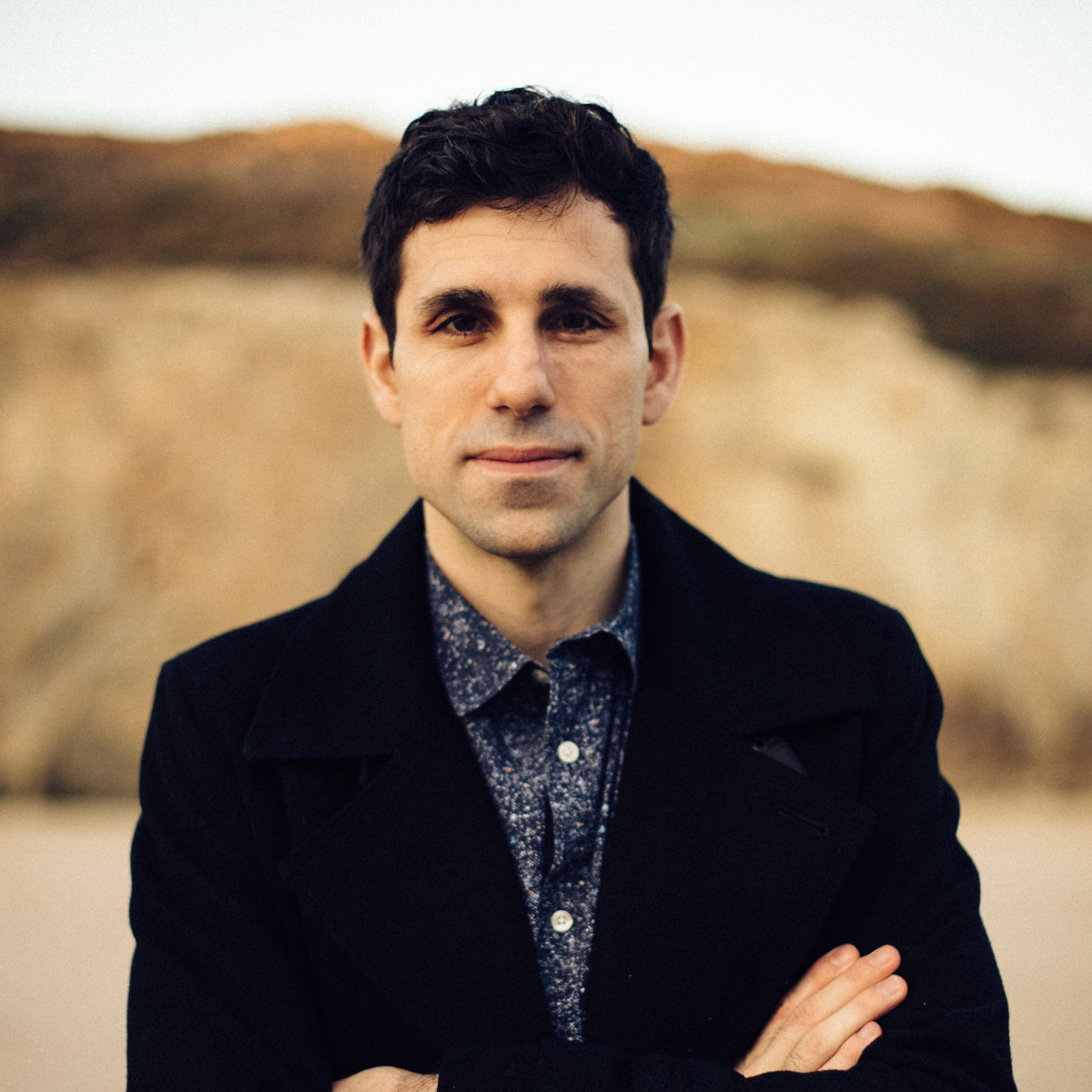
César de la Fuente is a Presidential Assistant Professor at the University of Pennsylvania, where he leads the Machine Biology Group. His research goal is to use the power of machines to accelerate discoveries in biology and medicine. Specifically, he pioneered the development of computer-designed antibiotics with efficacy in animal models, demonstrating the application of AI for antibiotic discovery and helping launch this emerging field. His lab has also been in the vanguard of developing computational methods for proteome mining and the first to find therapeutic molecules in extinct organisms, launching the field of molecular de-extinction. Additional advances from his lab include designing algorithms for antibiotic discovery, reprogramming venoms into antimicrobials, creating novel resistance-proof antimicrobial materials, and inventing rapid, low-cost diagnostic devices for COVID-19 and other infections.
César is an NIH MIRA investigator, receiving recognition and research funding from numerous other groups and over 60 national and international awards. Most recently, he was awarded the prestigious Princess of Girona Prize for Scientific Research, the ASM Award for Early Career Applied and Biotechnological Research, and the Rao Makineni Lectureship Award by the American Peptide Society and was selected as a National Academy of Medicine Emerging Leader in Health and Medicine.
César serves on the editorial boards of more than 20 scholarly journals and is currently an Associate Editor of Drug Resistance Updates (the premier international drug resistance journal), Nature Communications Biology, Bioengineering & Translational Medicine, and Digital Discovery.
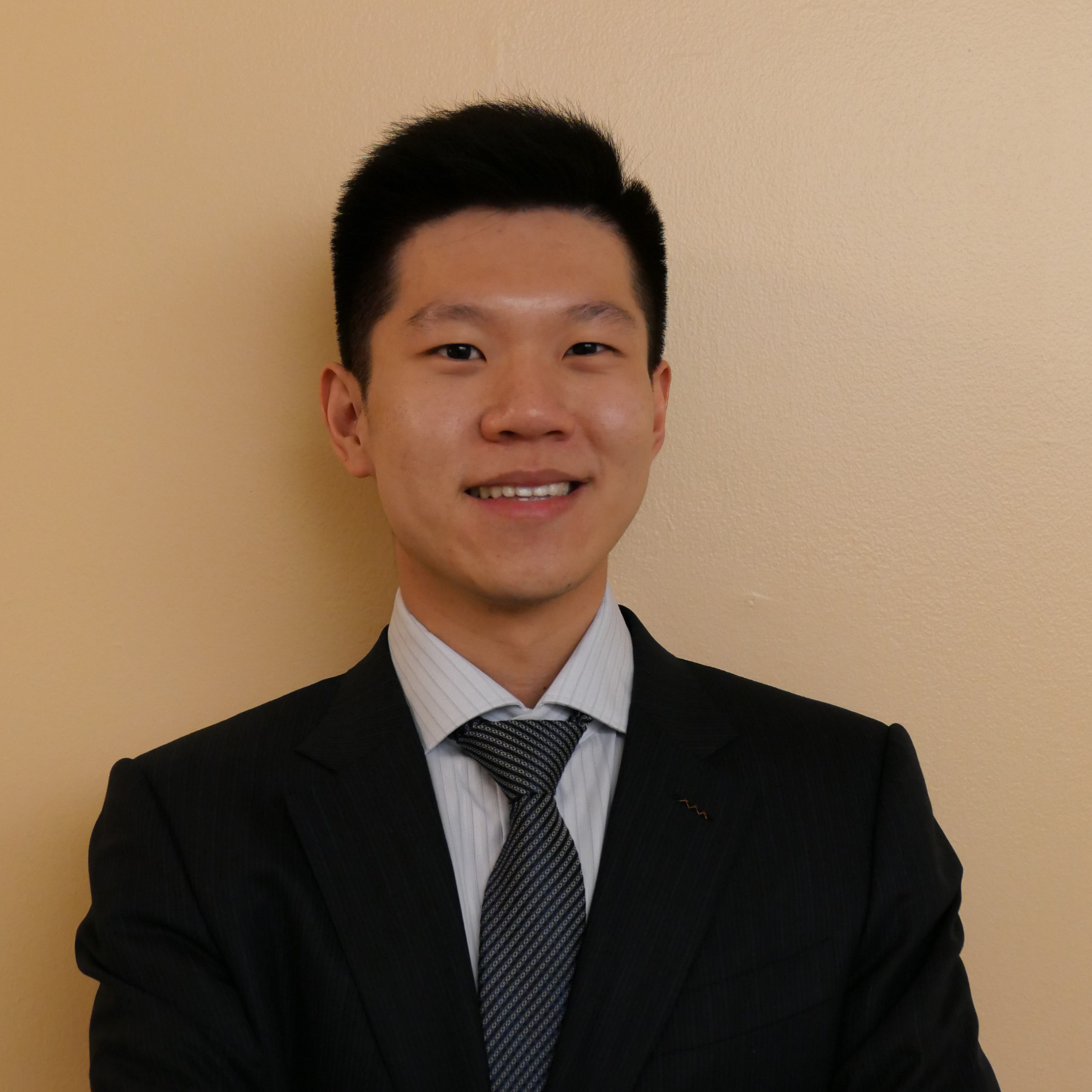
Shuangzhe Lin is pursuing a PhD degree in Biomedical Engineering at Rensselaer Polytechnic Institute (RPI). He is interested in engineering biomolecules to develop innovative therapeutics and tools to ultimately improve the quality of human life. He received his Bachelor of Science degree with High Distinction and High Honors in Biochemistry from the University of Michigan – Ann Arbor and, in 2023, obtained his Master of Biotechnology degree from the University of Pennsylvania.
In 2021, he joined Prof. Cesar de la Fuente-Nunez’s Machine Biology Group and worked on engineering commensal bacteria to deliver antimicrobial peptides (AMPs) into the gut to combat antibiotic-resistant pathogens and remodel local microbiomes. He also studied a subclass of AMPs that contain a metal-binding motif, known as the amino-terminal copper and nickel (ATCUN) motif, to design peptide antimicrobials against multidrug-resistant bacteria.
The authors declare that they do not have any relationships or affiliations that could be construed as a potential conflict of interest.
The views and opinions expressed in this article are solely those of the original author(s) and do not necessarily represent those of GARDP, their donors and partners, or other collaborators and contributors. GARDP is not responsible for the content of external sites.